AI in the insurance industry – terms and definitions
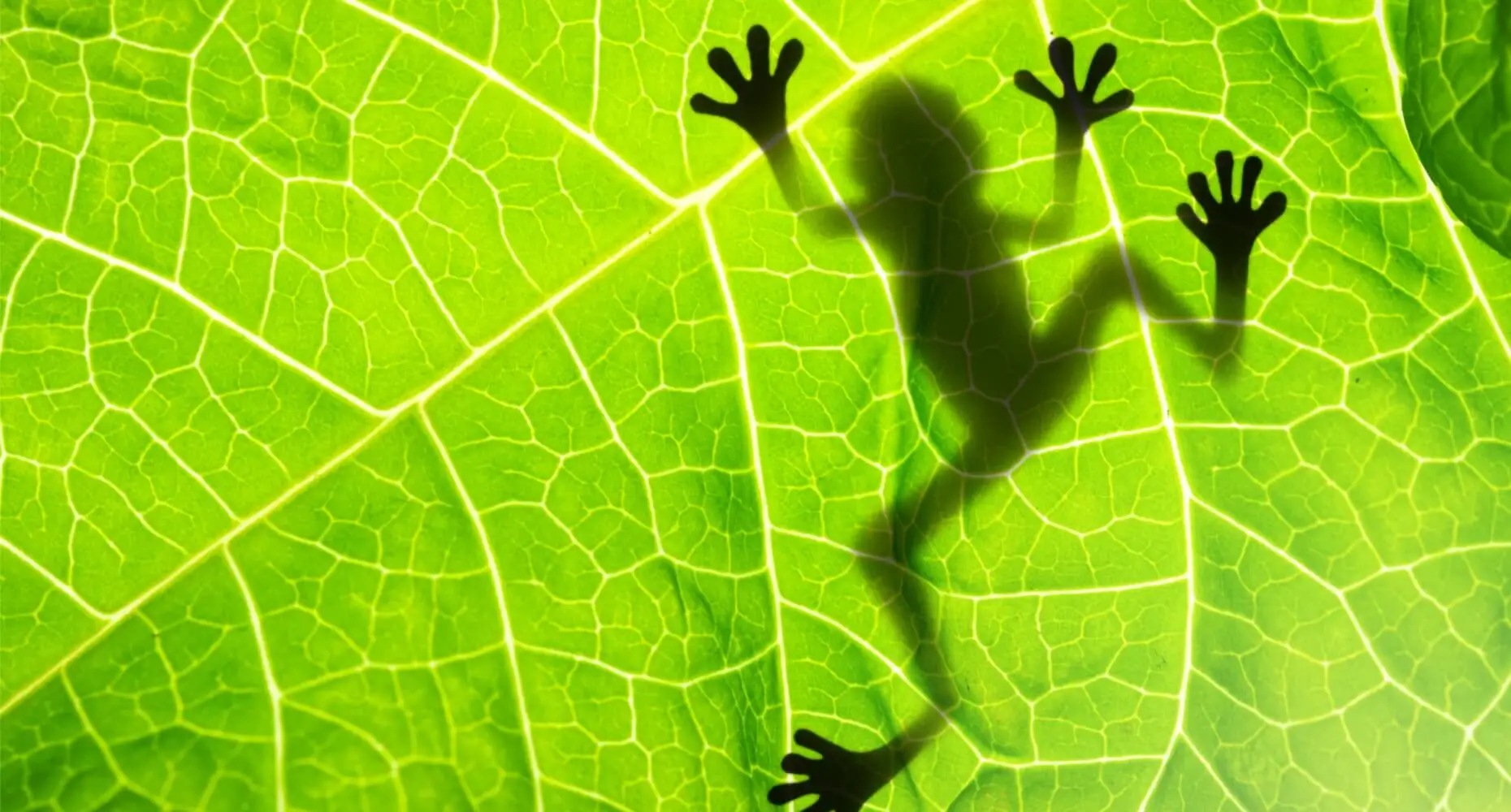
The media extols the virtually limitless possibilities that artificial intelligence (AI) offers on almost a daily basis. The insurance industry is no different, with one news story following another: AI applications track down insurance fraudsters by sifting through social media activity, analyse the musculoskeletal system using a filmed squat, or understand elements of the Bavarian language in customer complaints. To keep track of things and shed some light on the world of AI, we’d like to take a look at AI in the insurance industry in a series of blog posts.
While buzzwords such as ‘artificial intelligence’, ‘machine learning’ and ‘deep learning’ are used often, and sometimes even as synonyms, it’s rare that they’re explained in more detail. But it’s vital to define and distinguish the terms first of all before going on to present potential areas of application or use cases for AI in the insurance industry.
What’s the difference between AI, ML and DL?
Artificial intelligence is interpreted in very different ways. There’s no universally valid definition – probably also because science still disagrees on how exactly to describe ‘intelligence’. The word ‘intelligence’ comes from the Latin ‘intellegere’, meaning ‘to understand’, ‘to see’ and ‘to comprehend’.
The term ‘artificial intelligence’ originally referred to the attempt to have machines replicate human-like decision-making structures (usually with software). Specifically, the goal is to build a machine that is capable of independently performing tasks or solving problems. This allows computer programs to recognise rules and patterns based on data, learn from experience and optimise themselves.
The Dartmouth Summer Research Project on Artificial Intelligence organised by John McCarthy in New Hampshire in 1955 is considered the birth of artificial intelligence. In the course of the project, the term ‘artificial intelligence’ (AI) was accepted as the official name of a new research discipline. Before that, in 1950, the English mathematician Alan Turing had already developed a test to determine whether machines were capable of thought equal to that of a human being. During the test, a person holds a conversation with a human and a machine. If the questioner cannot clearly tell which is the machine and which is the human following intense questioning, the machine has passed the Turing test. Driven by almost unlimited access to computing power (cloud computing) and big data, AI has been experiencing a renaissance for several years.
Learning from data
Machine learning (ML) is a branch of artificial intelligence. ML is a method that contrasts with classic programming, where an algorithm (that is known and programmed in advance) is processed for data input. In the case of ML, an algorithm is not known beforehand (and usually not afterwards, either). Instead, a model is trained based on a learning method using the input data. It is also said that ‘the model learns from the data’. We come across ML applications everywhere in our everyday lives. Amazon’s product recommendations, Netflix’s movie recommendations, and the Siri or Alexa virtual assistants are just a few examples.
‘Machine learning’ is a generic term used to refer to a variety of learning methods that are split into different categories to provide a better overview. The most important of these categories are supervised learning and unsupervised learning. In the case of supervised learning, the learning algorithm is trained on examples with a known outcome, then used to make predictions when given new data. In other words, a machine learns to derive the outcome data from the initial data. In the case of unsupervised learning, there is no predictable outcome. The goal is to describe relationships and patterns between descriptive features. With unsupervised learning, intrinsic patterns are extracted from the data using different methods.
Deep learning is a special method of machine learning that is very popular at present and based on artificial neural networks (ANNs), particularly special ‘deep networks’. ANNs are structured based on the biological neurons of the human brain.
Artificial neural networks aren’t a new discovery. As early as 1943, Warren McCulloch and Walter Pitts described artificial neural networks of linked elementary units that could, in principle, compute any arithmetic or logical function. In 1958, Frank Rosenblatt introduced a concept for simple neural networks under the name ‘Perceptron’.
Data, data and even more data
ANNs are trained using data. With the help of machine learning, big data (i.e. the immense and constantly growing volumes of data created by the global spread of internet technologies) can be transformed into smart data (i.e. data whose valuable content has been tapped). The boom surrounding ANNs is mainly due to the immense increase in computing capacity, which enables highly efficient, fast processing and analysis of complex models.
The science that deals with the processing, analysis and presentation of data is called data science. This interdisciplinary field is essentially aimed at gaining insights from data that can be used as a basis for business decisions and forecasts. Statistical or ML methods are applied to mass data using appropriate computing infrastructures with the aim of answering subject-specific questions.
The terms ‘data mining’ and ‘data analytics’ refer to specific data analysis. This refers to the systematic application of machine learning and statistical methods to identify hidden relationships, patterns and trends in large data sets.
If you’re interested in how AI-based solutions are already being used in the insurance industry, be sure to stick around. Some use cases will be presented in the following blog posts.